The First AI Winter: When Early Promises Met Reality
The first "AI Winter," spanning roughly from 1974 to 1980, marks a significant period in the history of artificial intelligence research. It was a time characterized by a dramatic decline in enthusiasm, funding, and perceived progress, following an era of intense optimism during the 1950s and 1960s. During those initial decades, pioneers made bold predictions about the imminent capabilities of thinking machines, setting the stage for a subsequent wave of disillusionment when reality fell short.
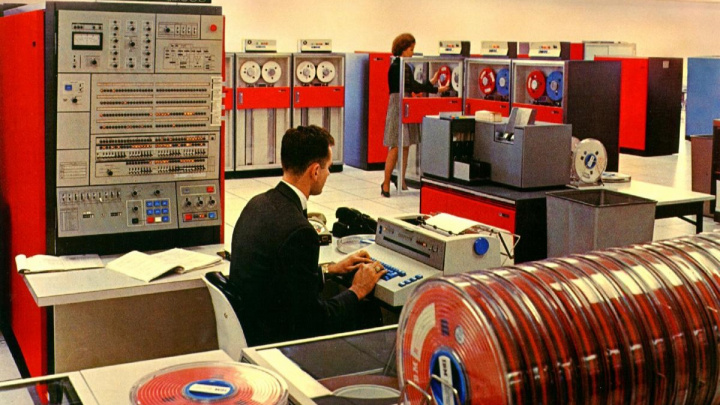
The onset of the first AI Winter wasn't due to a single event, but rather a confluence of factors. These included overly ambitious promises that couldn't be kept, fundamental limitations in the available technology and theoretical understanding, and influential critiques that questioned the very foundations and progress of the field, ultimately leading to severe funding cuts.
An "AI Winter" signifies a period marked by a significant reduction in interest and funding for artificial intelligence research. These periods are often characterized by cycles of excessive hype followed by disappointment when results fail to match expectations.
Key Factors Contributing to the First AI Winter
Several critical issues converged to trigger this downturn:
- Overblown Promises and Unmet Expectations: Early AI researchers, buoyed by initial successes in limited domains (like game playing or simple logic), made highly optimistic predictions. Figures like Herbert Simon famously predicted in the late 1950s and 1960s that machines would be capable of doing any work a man can do within twenty years. When these grand visions failed to materialize – machines couldn't understand natural language fluently, translate accurately, or exhibit general common-sense reasoning – disappointment grew among sponsors and the public.
- Fundamental Technical Hurdles:
- The Combinatorial Explosion: Researchers discovered that many seemingly straightforward problems became computationally intractable as the scale increased. Methods that worked for simple "toy" problems failed spectacularly on real-world tasks because the number of possibilities to explore grew exponentially, overwhelming the available computing resources.
- Insufficient Computing Power and Memory: The hardware of the era, while advancing, was simply not powerful enough to handle the complex calculations and large amounts of data required by more ambitious AI programs. Processing speed and memory capacity were major bottlenecks.
- Lack of Large Datasets: Unlike today's data-rich environment, researchers lacked the large, digitized datasets needed to effectively train and test AI systems, particularly for tasks like language understanding or image recognition.
- Theoretical Limitations (e.g., Perceptrons): While slightly predating the main winter, the 1969 book "Perceptrons" by Marvin Minsky and Seymour Papert mathematically demonstrated the fundamental limitations of simple, single-layer neural networks (the dominant connectionist model at the time). They showed these networks couldn't even solve basic problems like the XOR function, casting doubt on their potential for complex tasks and significantly dampening enthusiasm for neural network research for over a decade.
- Damaging Critiques and Funding Cuts:
- ALPAC Report (1966, USA): This report by the Automatic Language Processing Advisory Committee, commissioned by US funding agencies, delivered a highly critical assessment of machine translation research. It concluded that machine translation was slower, less accurate, and more expensive than human translation and saw no prospect of practical, high-quality translation in the near future. This led to drastic cuts in funding for machine translation projects in the US.
- The Lighthill Report (1973, UK): Commissioned by the British Science Research Council and authored by applied mathematician Sir James Lighthill, this report offered a pessimistic view of AI research in the UK. It argued that AI had failed to achieve its grand objectives and that its methods were inadequate for solving real-world problems due to issues like the combinatorial explosion. The report led to severe cuts in AI research funding across British universities, effectively initiating the AI winter in the UK.
- DARPA's Shift in Focus (USA): The US Defense Advanced Research Projects Agency (DARPA), a primary source of AI funding, became increasingly frustrated with the lack of concrete progress in areas like speech understanding (e.g., the Speech Understanding Research program at Carnegie Mellon University). In the early 1970s, DARPA shifted its funding strategy towards more directed, mission-oriented projects with clearly defined, short-term goals, cutting support for more exploratory, undirected AI research.
- Moravec's Paradox Emerges: Researchers began to observe what Hans Moravec later articulated: tasks easy for humans (like perception, mobility, pattern recognition) proved incredibly difficult for AI, while tasks difficult for humans (like complex calculations or logical deduction in constrained domains) were relatively easier for computers. This highlighted the profound challenge of replicating basic human sensory and motor skills.
Source: Wikipédia
Impacts of the Freeze
The first AI Winter had profound consequences for the field:
- Drastic Funding Reductions: Government agencies (like DARPA in the US and the SRC in the UK) and private investors significantly reduced or eliminated funding for AI research.
- Project Cancellations and Slowdown: Many ambitious AI projects were shut down, and overall research activity slowed considerably.
- Shift in Focus: Researchers often shifted their focus to more applied areas of computer science with clearer prospects for short-term results, or rebranded their AI work under different labels (e.g., pattern recognition, informatics) to secure funding.
- Loss of Talent: Some researchers left the field altogether due to the lack of funding and perceived lack of progress.
- Fostering Pragmatism: On the positive side, the winter forced the remaining researchers to adopt more realistic goals and rigorous methodologies. It encouraged work on more constrained problems and foundational areas like logic programming, knowledge representation, and exploring different reasoning mechanisms (like common-sense reasoning) that laid the groundwork for future advancements.
Key Figures and Their Roles During This Era
- Marvin Minsky & Seymour Papert: Co-founders of the MIT AI Lab. Their 1969 book "Perceptrons" critically analyzed early neural networks, contributing significantly to the decline in connectionist research that coincided with the first winter.
- Sir James Lighthill: Author of the influential 1973 Lighthill Report, which severely criticized UK AI research and led to major funding cuts there.
- Herbert Simon & Allen Newell: Early AI pioneers whose optimistic predictions about AI's capabilities in the 50s and 60s contributed to the initial hype cycle.
- John McCarthy: Coined the term "artificial intelligence" and developed the LISP programming language, which remained crucial for AI research despite the winter.
- Yehoshua Bar-Hillel: An early skeptic, particularly regarding the feasibility of fully automatic high-quality machine translation, whose arguments gained traction leading up to the ALPAC report.
- Roger Schank & Marvin Minsky: Later, at a 1984 AAAI conference, they explicitly warned of a looming "AI Winter" (referring to the potential *second* winter), drawing an analogy with the concept of a nuclear winter and popularizing the term to describe these downturns.
Thawing and Lessons Learned
The first AI Winter did not represent a complete halt, but rather a significant cooling and re-evaluation. Research continued in specific areas, often with less ambitious claims. The lessons learned – the need for vastly greater computing power, larger datasets, more sophisticated algorithms, and more realistic goal-setting – were crucial. While the development of expert systems began to gain traction towards the end of this period and into the early 1980s (leading to a subsequent boom and eventually the second AI Winter), the end of the first winter was more of a gradual thaw. It was characterized by a more cautious, focused approach that slowly rebuilt credibility and paved the way for the eventual resurgence of different AI paradigms, including the renewed interest in machine learning and neural networks decades later, fueled by the very computational power and data that were lacking in the 1970s.